Researchers discover burstiness and strong memory combination in cyber intrusions
NewsAugust 01, 2017
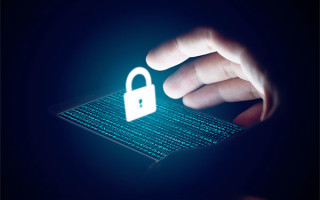
ADELPHI, Md. U.S Army Research Laboratory (ARL) researchers discovered that the process of intrusion detection in computer networks exhibits a significant degree of burstiness as well as strong memory.
Dr. Alexander Kott, an ARL Chief Scientist, and former colleague Dr. Richard Harang, who now works for a security software and hardware company Sophos Group PLC, found the burstiness, but they can't say for sure what causes it.
Kott explains that the combination of burstiness and strong memory suggests to cyber researchers that the reason behind the burstiness has something to do the threshold phenomena.
Burstiness and memory parameters suggest that the nature of the process under considerations is more reminiscent of natural processes such as earthquakes than of anthropogenic processes such as sending emails. Unlike anthropogenic processes, intrusion detection exhibits strong memory. To explain these findings, researchers propose the hypothetical mechanism called the Analyst Knowledge Threshold.
"The likely mechanism of the burstiness in intrusion detection is reminiscent of the integrate-and-fire, or similar threshold phenomenon: the analysts' knowledge about a new malware accumulates to the point until it becomes actionable and enables analysts to recognize a particular type of intrusion that was previously difficult or impossible to find. At that point, the analysts are able to rapidly recognize a number of pre-existing intrusions within a network under their care and produce multiple reports in rapid succession," Kott says.
Researchers say significant burstiness means that bunches ( or bursts) of events cluster together, occurring rapidly one after another, with much higher probability than if it was merely random, hinting that there is some underlying reason is behind that burst.
However, researchers do not conclude that burstiness is always present, in a general case, or can be observed in all networks and in all intrusion detection processes. In other words, they only show that burstiness is present in some cases, but not necessarily in all generic cases.
In their scientific paper ("Burstiness of Intrusion Detection Process: Empirical Evidence and a Modeling Approach”), researchers also demonstrated that higher burstiness is probably associated with higher percentage of sophisticated, hard-to-detect intrusions. That means, by looking at burstiness, it may become possible in the future to assess the sophistication of threats, and the degree of risk that the particular network is facing.
Their research does provide an analysis and a way of delivering a model to provide better understanding of the observed burstiness. It also provides open opportunities for quantifying a network's risks and requirements for defensive efforts. This is the first demonstration that involves data from multiple organizations and use of several statistical techniques, of burstiness in the process of detecting infections effected by cyber threats against networks of large organizations.
"To the best of our knowledge, this is the first demonstration, with strong empirical evidence that involves data from multiple organizations and use of several statistical techniques, of burstiness in the process of detecting infections effected by cyber threats against networks of large organizations," Harang says.
Companies tend to use a Managed Security Service Provider (MSSP) to monitor computer networks and analyze the information obtained. When MSSP detects intrusions and activities of malware on the network, it makes sure to confirm that it is a real intrusion and then reports such detections to the operators of the network. Those, in turn, take measures necessary to recover from the intrusion. MSSP analysts strive to submit each report as soon as possible after detecting an infection. Thus, the process of monitoring and analysis yields a series of incident reports each of which documents a detection of an infection.
"We looked at empirical data provided to us by a MSSP covering several large, non-residential networks, belonging to several organizations,"Harang says. These were essentially time series of intrusion reports. First, we used several statistical techniques to determine whether the timing of those reports is random, that is complying with the so-called Poisson process."
Researchers found that when they apply the statistical technique called Kolmogorov test to the arrival and inter-arrival times of the time series, the test shows that at least for some of the organizations, this distribution clearly violates a Poisson process. Examining a variant of the K-statistic across a range of scales shows that the rate at which observations cluster is significantly higher than that of the Poisson process.
Finally, researchers estimated memory and burstiness parameters of the data, and found that some data sets marked deviations from the Poisson process. Although the burstiness is less pronounced in some cases, it is highly visible in the largest datasets that cover the largest number of intrusions.
ARL researchers developed a way to model and simulate this burstiness in response to this discovery, which managers can use in cyberdefense organizations to estimate how variable would be the workload cyber analysts face over time. "With that, they can make appropriate arrangements for workforce scheduling, arranging for surge capacity and the like."
Their scientific paper "Burstiness of Intrusion Detection Process: Empirical Evidence and a Modeling Approach,” is scheduled for publication in the journal IEEE Transactions on Information Forensics and Security in October 2017.