Performance analysis tools developed for IARPA's insider threat program
NewsDecember 06, 2017
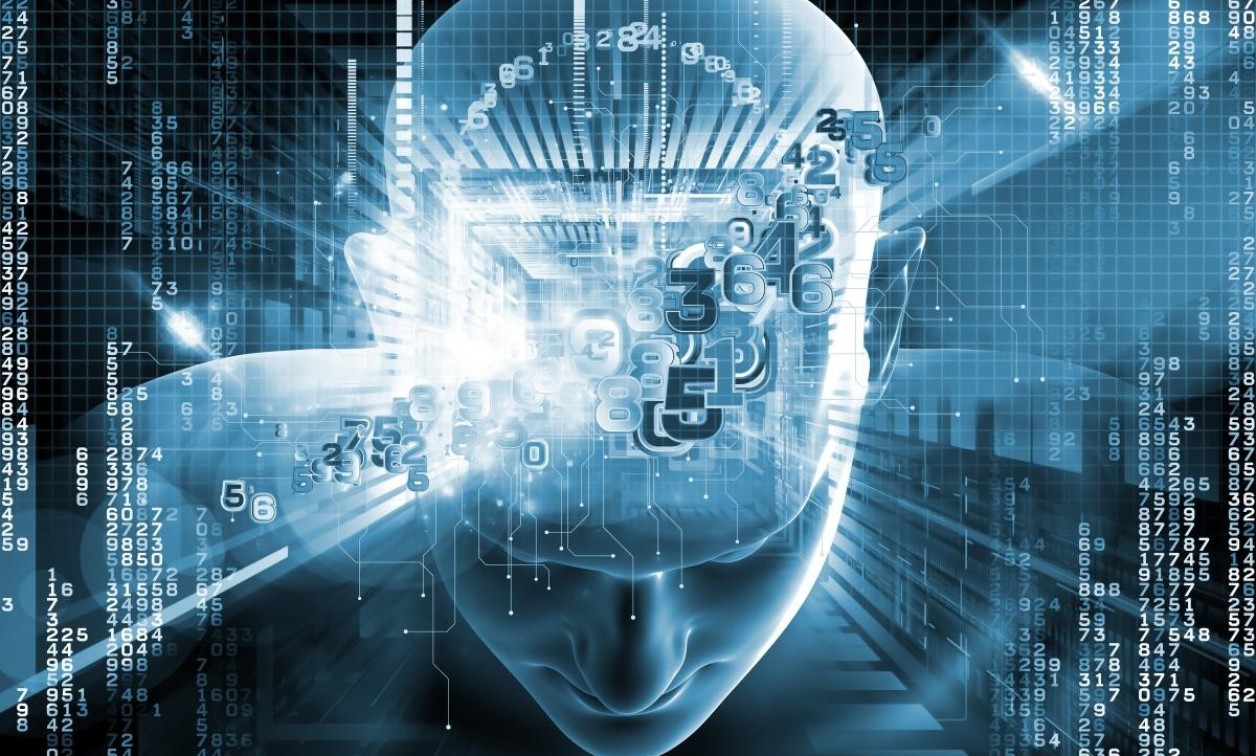
CAMBRIDGE, Mass. Engineers at Charles River Analytics developed prediction and sensitivity analysis tools and algorithms under the Continuous Insider Threat Evaluation (SCITE) program for the Intelligence Advanced Research Projects Activity (IARPA).
To achieve the goal of the program, Charles River led a team under the Probabilistic Relational Inference Modeling for Enterprises (PRIME) effort, which resulted in probabilistic models of enterprise-deployed machine learning systems (such as insider threat detection systems). The team included Assured Information Security, Applied Marketing Science, and Cognitio.
Analysts can use these models to evaluate, forecast, and understand the performance of a machine learning system within the enterprise.
The SCITE program sought to develop and test methods to detect insider threats - individuals with privileged access within an organization who are, or intend to be, engaged in malicious behaviors such as espionage, sabotage or violence - through two separate research thrusts, according to IARPA. The first research thrust will develop a new class of insider threat indicators, called active indicators, where indicative responses are evoked from potential insider threats. The second research thrust will develop Inference Enterprise Models (IEMs) that forecast the accuracy of existing and proposed systems for detecting insider threats.
Read more on machine learning:
Team investigates artificial intelligence, machine learning in DARPA project
The role of machine learning in autonomous spectrum sharing
Cognitive electronic warfare: Countering threats posed by adaptive radars